Annotating cells in the Tabula Muris Senis mouse liver dataset (CellKb vs CellTypist)
10 October 2024
In this article, we use CellKb to assign cell types to individual cells in the mouse liver dataset from the Tabula Muris Senis publication.
This dataset contains 7,294 single cells and is available on the cellxgene website
here.
Author annotations
The cell types provided by the authors in the original publication and available through cellxgene. Hepatocytes have been annotated at a higher level of granularity. However, other cell types, immune cells in particular are assigned broad or mixed cell type annotations.
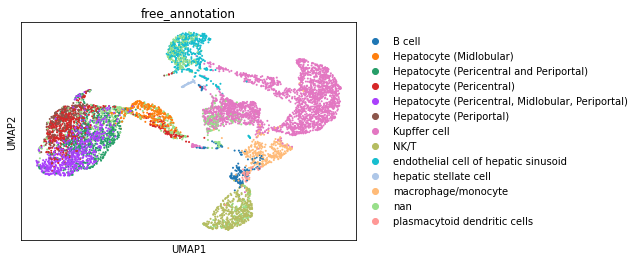
Cell type annotation with CellKb
We used the steps given in our previous blog here to upload, process and annotate the scRNA-seq data in h5ad format. We chose "Mouse" as the species and "Liver" as the anatomical structure in the filter criteria.
The UMAP belows show the cell types predicted by CellKb in this dataset using a reference dataset of 1,049 marker gene sets representing 176 cell types from 37 publications. Due to the large number of reference cell types available, CellKb is able to predict cell types
at varying levels of granularity. Specifically, CellKb correctly identifies the region specific hepatocytes found in the liver - periportal, centrilobular and midzonal. CellKb is also able to provide greater granularity for the cell type originally assigned as "NK/T" cells.
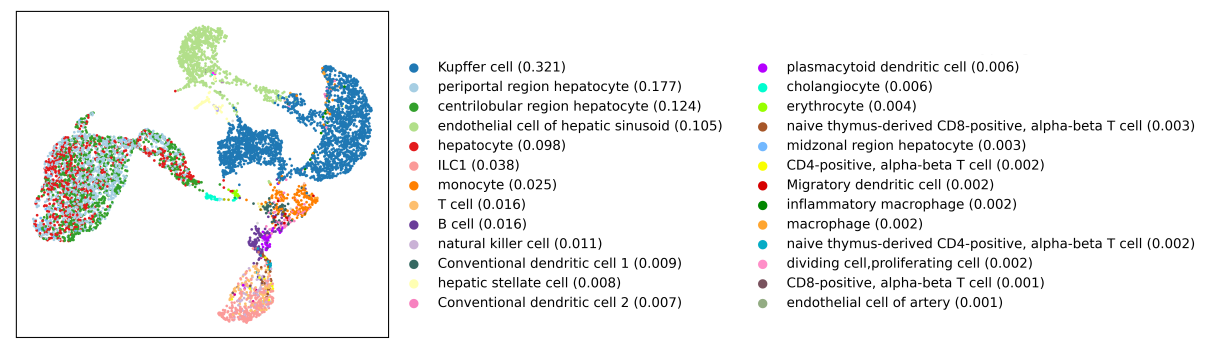
Cell type annotation using CellTypist
We analyzed the same mouse liver dataset using scanpy with CellTypist using a precalculated model of healthy mouse liver.
As seen in the UMAP below, CellTypist is able to identify the broad cell types in this mouse liver dataset. CellTypist predicts the granular cell type annotations for some immune cell types, though it fails to assign monocytes and macrophages separately. It is also not able to identify the region specific hepatocytes due to the limited number of cell types available in the reference model.
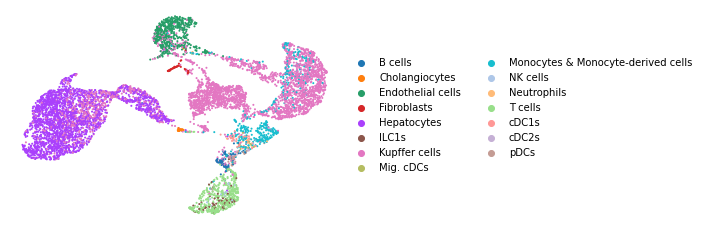
We share our python code here:
import celltypist
from celltypist import models
import pandas as pd
import scanpy as sc
models.models_description(on_the_fly = True)
# cellxgene mouse liver 10x
adata = sc.read("./tabulamurissenis/liver/mouse_liver_10x.h5ad")
adata1 = adata.raw.to_adata()
adata1.layers["counts"] = adata1.X.copy()
sc.pp.normalize_total(adata1)
sc.pp.log1p(adata1)
sc.pl.umap(adata1, color='cell_type')
sc.pl.umap(adata1, color='free_annotation')
adata1.var.index = adata1.var['feature_name']
predictions = celltypist.annotate(adata1, model = 'Healthy_Mouse_Liver.pkl')
adata1.celltypist = predictions.to_adata(insert_labels=True, insert_conf=True, insert_decision=True)
sc.pl.umap(adata1.celltypist, color="predicted_labels", title="", frameon=False)
# cellxgene mouse liver smartseq
adata = sc.read("./tabulamurissenis/liver/ss/mouse_liver_ss.h5ad")
#adata1 = adata.raw.to_adata()
#adata1.layers["counts"] = adata1.X.copy()
sc.pp.normalize_total(adata)
sc.pp.log1p(adata)
sc.pl.umap(adata, color='cell_type')
sc.pl.umap(adata, color='free_annotation')
adata.var.index = adata.var['feature_name']
predictions = celltypist.annotate(adata, model = 'Healthy_Mouse_Liver.pkl')
adata.celltypist = predictions.to_adata(insert_labels=True, insert_conf=True, insert_decision=True)
sc.pl.umap(adata.celltypist, color="predicted_labels", title="", frameon=False)
Conclusion
Compared to CellTypist, CellKb is able to identify cell types at greater granularity because it contains a large reference dataset of cell types from multiple publications. CellKb also uses standardized ontology terms in the results making it easier to integrate into existing pipelines.