Get, set, annotate
How AI cell type annotation methods compare to traditional approaches
21 April 2025
Single-cell RNA-sequencing has revolutionized the study of biological systems. Individual experiments now generate gene expression profiles of hundreds of thousands of cells
while integrated organ atlases combine millions of cells from multiple experiments. This data is then used to identify the cell types in organs and the role they play in normal and disease conditions.
Cell type annotation or the assigning of cell types to cells is an important part of scRNA-seq data analysis.
In general, there are two types of cell type annotation methods - manual and automated. Automated methods can be further divided into marker-based
methods and reference-based methods. These are covered in detail in single-cell best practises.
With the rapid advances in AI, there has recently been a surge of cell type annotation methods using foundation models.
In this blog, we explore some of the recent approaches of cell type annotation using foundation models and compare them with traditional methods.
We find that traditional methods are, in general, easier to setup and use compared to AI methods. They also have comparable or better performance. Annotation quality across all methods
depend on the availability of a suitable reference dataset with high-quality annotations, either for training AI models or searching and reference mapping in traditional methods.
The table below summarizes our findings.
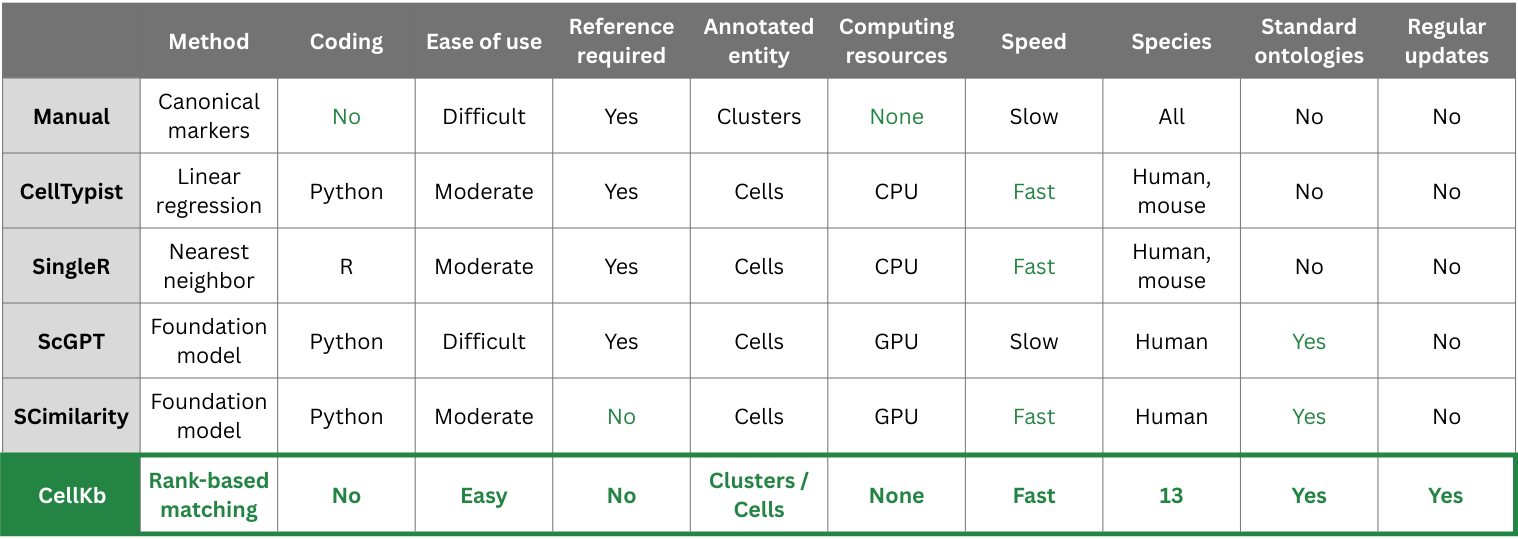
Data and methods used in the blog
We used data from the Asian Immune Diversity Atlas (AIDA), specficially
AIDA v2, which was downloaded in h5ad format from CellXGene.
We randomly selected 10% of the cells from each cell type cluster for analysis. We installed and ran each method as per the instructions given on their websites following the published tutorials.
A total of 126,546 cells were annotated using the methods described below. We then compared the predicted annotations with the manual annotations, checking the percentage of exact matches recovered
by each method. For the comparison of predicted cell types, we used the cell ontology terms from CellXGene. A cell type prediction was considered correct if the predicted Cell Ontology
term exactly matched the manual annotation, or was a direct parent or child of it.
1. Traditional methods for cell type annotation
Traditional methods are those that have been used for cell type annotation prior to the development of those based on foundation models.
These can further be divided into two major groups.
1.1 Manual annotation
- This method is time and effort intensive since it requires manual checking of canonical marker expression in cells.
- It involves clustering the data into groups of similar cells and calculating the cluster-specific gene lists.
- Genes that show statistically significant upregulation in a cluster compared to other clusters can then be used to assign a cell type to that cluster.
- Upregulated genes are compared manually to known canonical markers or previously identified cell type specific marker genes.
- Some databases like CellMarker and PanglaoDB are also used to find known marker genes for cell types. (Read our blog on the comparison of CellKb with PanglaoDB).
- The annotations obtained by this method can be highly reliable if they are meticulously assigned.
Pros
- Complete control over annotations
- Based on published literature with link to reference
- Reliability varies with publication
Cons
- Requires known cell type specific marker genes
- Time-consuming since references or databases with canonical markers have to be manually searched
- Requires accurate clustering (merging multiple cell types or loss or rare cell types during clustering)
- Public marker gene databases are not regularly updated
- Standardized ontology terms are not used
Example: Manual annotation of immune cell clusters in the AIDA dataset
assigned by the authors based on canonical markers.
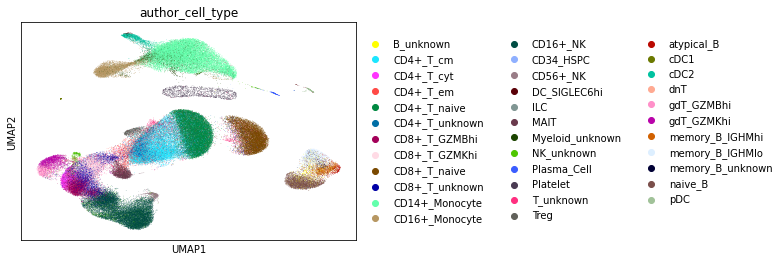
1.2 Automated annotation
- These methods involve classification or reference mapping algorithms to automatically assign cell types to individual cells.
- They require a single high-quality reference dataset or a suitable reference of multiple integrated datasets that is very similar to the query dataset.
- These methods can provide accurate annotations if the reference data used contains a sufficient representation of the cell types in the query dataset and is generated using the same methods as the query.
- Reliable annotation prediction requires annotating against multiple references individually or combined, since reference datasets that closely match the query datasets are not always available.
- Installation of an R or Python library along with a previously trained machine-learning model to be used as reference is needed.
- Cell type prediction is done for individual cells removing the need for clustering.
Pros
- Assign cell type to each cell so clustering is not needed
- Provide reliable annotations if a matching reference dataset in terms of content and experimental technique is available
- Faster than manual annotation
Cons
- Matching reference datasets are not always available
- Creating a custom reference dataset by integrating single-cells from multiple datasets is non-trivial due to batch effects
- Customization of trained model is not possible
- Require installation and programming in Python or R
- Models are rarely updated
- Standardized ontologies are not always used
Examples: CellTypist, SingleR, Azimuth, scArches. Check out our blogs comparing the performance of CellTypist and SingleR with CellKb
here and here.
CellTypist Prediction
The UMAP below shows the cell types predicted by CellTypist. CellTypist
uses a logistic regression classifier optimized by the stochastic gradient algorithm for automated cell type annotation.
It provides pre-trained models for multiple organs in human and mouse. We used Immune_All_Low model for cell type prediction. Since CellTypist does not provide the
annotations in standardized cell ontologies, we manually mapped the predicted cell types to Cell Ontology terms. CellTypist predictions for 82,802/126,546 (65.4%) cells matched the author annotations.
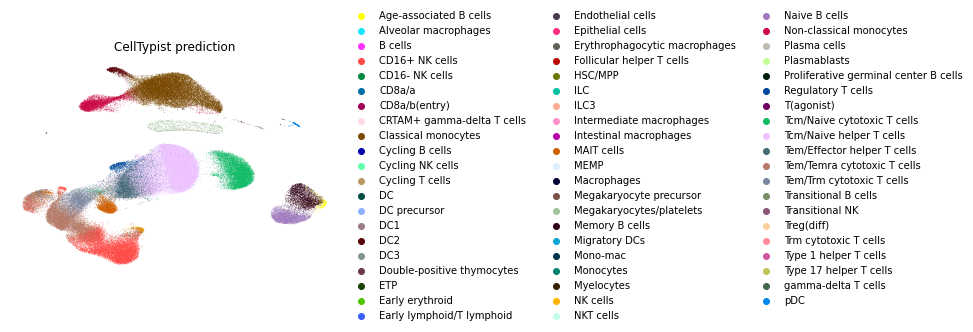
2. AI annotation methods
- These methods use foundation models trained on millions of cells. Several methods have been recently developed.
- They annotate individual cells and hence do not require the data to be clustered.
- They can be run with or without a reference dataset, though some methods work best when their model is fine-tuned using a suitable reference.
- Since the model is pretrained they offer no way to customize the reference data in the model.
- The models are also not regularly updated since they take significant amount of time and computational resources for training.
- They are often difficult to set up requiring installation of multiple Python libraries in custom system environments and, in most cases, a GPU.
- Speed can vary depending on the requirement of fine-tuning.
Pros
- Can work without a reference
- No clustering needed
- Multiple references are integrated into the model
- Faster than manual annotation
Cons
- Customization of trained model is not possible
- Trained models are infrequently updated
- Require difficult and time-consuming installation and programming in Python
- May require GPU to execute
- Some zero-shot models are not very accurate, need reference for fine-tuning
- Models are not frequently updated
Examples:
Scimilarity, scTab, scGPT, Geneformer
3. CellKb cell type annotation
- CellKb is a knowledgebase of high-quality cell type signatures collected from manually curated reference publications in literature.
- CellKb provides a web-based interface that allows users to specify cell type signatures from thousands of publications as reference.
- Multiple references can be used together without the need for integration.
- It can be used to annotate individual cells or clusters.
- It uses a fast and robust rank-based search method to find matching cell types signatures in the knowledgebase and predict cell types.
- It does not require installation of libraries or searching for a suitable reference.
- The reference database in CellKb is updated every 3 months with the cell type signatures from the latest publications.
Pros
- No need to search for a suitable reference since CellKb comes with a knowledgebase
- Multiple references selected and used individually without need for integration
- Fast
- No installation or programming required
- Regular updates
- Annotates clusters or cells
Cons
- Not a free service
4. Final thoughts
Overall, both traditional and AI methods seem equally capable of identifying most cell types, at least in the case of the AIDA dataset.
Manual annotation is often time-consuming, as it involves consulting a vast and ever-growing body of literature—over 50,000 single-cell studies were published between 2013 and 2024.
However, using AI methods also comes with limitations. It requires significant computationational resources, installation and coding.
Installing and running scGPT is particularly challenging and all available models have not been recently updated.
Foundation models like zero-shot scGPT and SCimilarity also do not allow for organ-level subsetting or selection of reference data.
Finally, AI methods perform well for cell type that are well-represented in training data such as immune cells, but struggle with rare or tissue-specific cell types with insufficient training data.
The table below summarizes the key features of each approach.
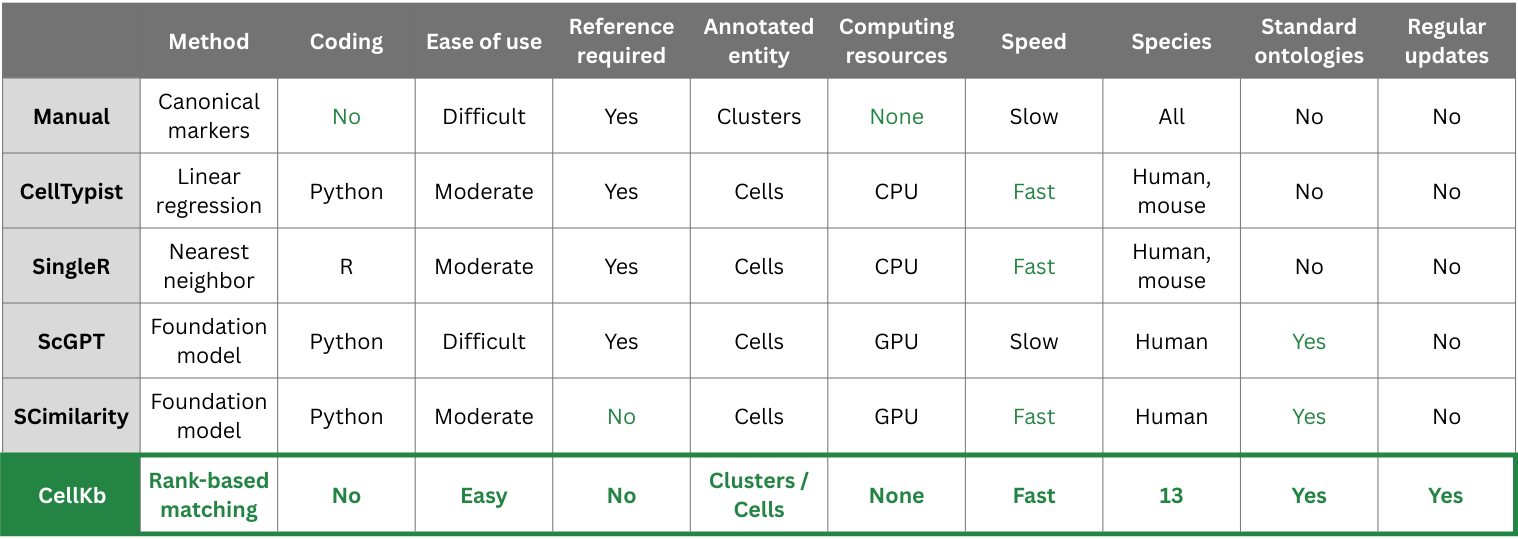
Thus, despite the wide range of available methods, accurately annotating cell types in scRNA-seq data remains a challenge.
CellKb address this challenge by providing a single, high-quality reference database of cell type–specific marker genes that is comprehensive and regularly updated along with an accurate cell type prediction method and a user-friendly web application,
significantly reducing the burden of cell type annotation in single-cell RNA-seq analysis.
Note: In this article, we have selected methods that work with a pre-existing reference or model.
Evaluation was based on the overlap between Cell Ontology terms in AIDA and those predicted by each method. The performance of each method is specific to the AIDA v2 dataset and may vary with different data or references.
It is possible that scGPT and SCimilarity were trained on AIDA,
potentially inflating their accuracy. Additionally, the manual AIDA annotations which are used as the gold standard here are assigned at cluster level rather than for individual cells.
Therefore, comparing predicted ontologies to AIDA annotations may not be optimal unless the predictions are also cluster-based, as with CellKb cluster-based annotation.